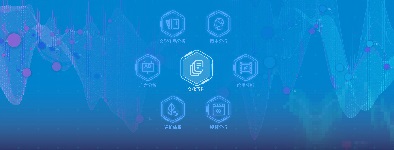
你的 AI 技能正在贬值!
我们正处于人工智能的繁荣时期,机器学习专家的薪水高得惊人,投资者与人工智能初创企业会晤时,往往乐于敞开心扉,慷慨解囊。
这么做是正确的:因为这是每一代都会发生一次的变革性技术之一,AI这项技术将会继续存在,改变我们的生活。
但这并不意味着让你的人工智能创业获得成功是一件容易的事。
我认为,任何人在试图围绕AI开展业务之前,都会遇到一些重要的陷阱。
你的 AI 技能正在贬值
2015 年我还在 Google 工作,那会儿就开始鼓捣 DistBelief(后来改名为 TensorFlow)。这玩意儿那时候实在太槽糕了,写起来非常笨拙,主要的抽象还不符合你的预期。
要想让它在 Google 所构建的系统之外发挥作用?
那真是一个白日梦。
儿子与我,使用艺术风格迁移进行图像处理,这项技术激发了我对深度学习的兴趣
2016 年底,我正在进行一个概念证明的研究,就是在组织病理学图像中检测出乳腺癌。我想使用迁移学习:采用 Inception,这是 Google 当时最好的图像分类架构,然后使用我的癌症数据重新进行训练。我使用了 Google 提供的经过预训练的初始权重,只不过更改了顶层以便能够匹配我所做的工作。我在 TensorFlow 中,经过长时间的反复实验后,终于弄明白了如何操纵不同的层,并使其大部分发挥作用。这些都需要很大的毅力去阅读 TensorFlow 的资料,但至少我不必太过担心依赖关系,因为 TensorFlow 准备好了 Docker 镜像,真是太贴心了!
在 2018 年初,由于缺乏复杂性,上述任务并不适合作为实习生的第一个项目。多亏了 Keras(TensorFlow 之上的一个框架),你只需几行 Python 代码就可以完成,而且不需要深入理解你在做什么。但有一个痛点,就是超参数调优。如果你有深度学习模型,那你可以调整多个参数,如层的数量和大小等等。但如何得到最优配置并非易事,一些直观的算法(如网格搜索)效果并不怎么样。你做了很多实验,感觉更像是一门艺术,而不是一门科学。
在我写下这些文字的时候(2019 年初),Google 和 Amazon 已经提供了自动模型调优服务(Cloud AutoML、SageMaker),Microsoft 也正在计划提供这一服务。我预测,手动模型调优将会像渡渡鸟一样灭亡,而对于机器学习工程师来说,这也算是一种很好的解脱。
我希望你们能明白其中的规律:困难的事情终将变得容易,你可以在获得更多的同时而无需深入理解。过去那些伟大的工程壮举,现在听上去却有些蹩脚。因此,我们不应该期望现在的壮举在未来会变现得更好。
这是一件好事,也是取得惊人进步的标志。我们将这一进步归功于像 Google 这样的公司,正是它们在这些工具上投入巨资,然后免费给人们提供这些工具。它们之所以这样做,主要有两个原因。
你被商品化之后的办公室
第一个原因,这是对它们实际产品(即云基础设施)的商品化补充的尝试。在经济学中,如果你倾向于一起购买两种商品的话,那么购买的这两种商品往往是互补的。举一些例子:汽车与汽油、牛奶与谷物、培根和鸡蛋等。如果其中一种商品降价了,那么另一种商品的需求往往就会增加。对云计算来说,它的补充就是运行在云端之上的软件;而人工智能有一个特点,就是需要大量的计算资源。因此,尽可能降低开发成本是很有意义的。
Google 如此热衷人工智能的第二个原因是,与 Amazon 和 Microsoft 相比,Google 拥有比较明显的优势:起步更早。毕竟是 Google 普及了深度学习的概念,因此,它们成功抢走了很多人才。它们在开发人工智能产品方面有着更多的经验,这些使得它们在开发必要的工具和服务方面占据了优势。
尽管取得了令人兴奋的进展,但对于那些在人工智能技能上投入巨资的公司和个人来说,并不是什么好消息。现在它们为你提供了坚实的竞争优势,因为培养一个称职的机器学习工程师,需要耗费大量的时间来阅读论文,以及打下扎实的数学基础。
然而,随着工具的改进,情况就不再如此:它将会变为更多的是阅读教程而不是科学论文。
如果你没有很快意识到自己的优势,那么图书馆的一群实习生可能就会抢走你的饭碗。特别是当实习生有更好的数据,这就引出了我的下一个观点……
更多的数据比花哨的 AI 架构更重要
假设你认识两个人工智能初创公司的创始人:Alice 和 Bob。他们的公司筹集到的资金大致相当,而且在同一个市场上激烈竞争。Alice 在最好的工程师和拥有丰富的人工智能研究经验的博士上进行投资,而 Bob 则雇佣了平庸但能干的工程师,并投资给她 (“Bob” 是 Robreta 的简称!)以获得更好的数据。那么,你会在哪个公司身上下注呢?
我会在 Bob 身上下注。为什么呢?
因为,从本质上来说,机器学习的工作原理就是通过从数据集中提取信息并将其传递给模型权重中。在这一过程中,更好的模型会更有效(就时间和(或)综合质量而言),但是假设某个足够的基线(即模型实际上正在学习某些东西),拥有更好的数据将会胜过更好的架构。
为了说明这一点,让我们做一个快速而粗略的测试。我创建了两个简单的卷积网络,其中,一个是 “更好” 的网络,一个是 “更差” 的网络。那个 “更好” 模型的最后一层全连接层 (Dense Layer) 有 128 个神经元,而 “更差” 的模型则只有 64 个。我在 MNIST 数据集的不断增大的子集上对这两个模型进行训练,并绘制出了模型在测试集上的正确率与训练的样本数的关系图。
蓝色曲线代表 “更好” 的模型,绿色曲线代表 “更差” 的模型
训练数据集大小的积极作用是显而易见的(至少在模型开始出现过拟合和正确率达到稳定之前是这样)。代表 “更好” 模型的蓝色曲线明显优于代表 “更差” 模型的绿色曲线。然而,我想要指出的是,就正确率而言,在 4 万个样本上训练的 “更差” 模型的表现,要比在 3 万个样本上训练的 “更好” 模型更好!
在我这个小例子中,我们处理的是一个相对简单的问题,并且我们还有一个全面的数据集。然而在现实生活中,我们可没有这么奢侈的条件。在许多情况下,你永远也无法避免图表中增加数据集具有如此显著效果的部分。
而且,Alice 的工程师们实际上不仅仅是与 Bob 的工程师竞争。由于人工智能社区的开放文化及其对知识共享的重视,他们还与 Google、Facebook、Microsoft 和全球数千所大学的研究人员竞争。
如果你的目标只是解决问题(而非对科学做出原创贡献),那么采用目前文献中描述的表现最好的架构,并根据你自己的数据对其进行重新训练,这是一个经过实战考验的策略。如果现在没有什么可用的东西的话,通常只需等待一两个季度,直到有人提出解决方案。特别值得一提的是,你可以做一些事情,比如举办一场 Kaggle 竞赛来激励研究人员研究你的特定问题。
良好的工程设计始终很重要,但如果你做的是人工智能的话,那么数据就是形成竞争优势的关键因素。然而,最重要的问题是,你是否能够保持住自己的优势。
保持 AI 竞争优势是很困难的
凭借出色的数据集,Bob 成功地与 Alice 展开竞争,她做得很好:推出了自己的产品,市场份额稳步增长。她甚至可以开始聘用更好的工程师,因为坊间传言她的公司是合适的选择。
Chunk 想要赶进度,不过他比 Bob 有更多的钱。这一点在构建数据集时很重要。通过砸钱来加速一个工程项目是非常困难的。事实上,指派太多的新人反而有可能会阻碍项目的进展。然而,创建数据集却是另外一种问题。通常来说,创建数据集需要大量的人工劳动,但你可以通过雇佣更多的劳动力来轻松扩展规模。或者可能某人拥有数据,那么你只需做的事就是向他支付许可费用。无论如何,有钱就是好办事。
那么问题来了,为什么 Chunk 能够比 Bob 筹到更多的资金呢?
当创始人发起新一轮融资时,他们会试图平衡两个可能存在冲突的目标。他们需要筹集到足够的资金才能胜出。但是,他们又不能筹集太多的资金,因为这样以来就会导致公司股权被过度稀释。接受外部投资者就意味着出售公司的一部分。创始团队必须在初创公司中保持足够份额的股份,以免他们失去创业的动力(要知道,创业可是一项艰苦的工作!)
另一方面,投资者也希望他们的投资是投在有着巨大潜力的点子上,但他们必须控制风险。随着感知风险的增加,他们会要求公司为他们支付的每一美元提供更多的股票份额。
当 Bob 筹集资金的时候,这是信心上的一次飞跃:人工智能能够真正提升她的产品。不管她作为创始人的素质如何,也不管她的团队有多优秀,毫无疑问,她一直在努力攻克的问题难以解决。而 Chunk 的情况非常不同,他知道这个问题是很容易解决的,因为 Bob 的产品就是活生生的证据!
Bob 应对这一挑战的可能反应之一是发起另一轮新的挑战。她应该处于有利地位,因为(目前)她在这场竞赛中仍然保持领先。然而,情况可能会更复杂。如果 Chunk 可以通过战略关系确保能够对数据的访问呢?遇到这种情况该怎么办?例如,假设我们正在讨论一家癌症诊断初创公司,那么 Chunk 可以利用他在一家重要医疗机构的内部地位,与该机构达成私下交易,而 Bob 很可能无法做到这点。
你的产品应该是可防御的,最好是有一条很深的护城河
那么,你将如何为人工智能产品构建可维护的竞争优势呢?
前段时间我有幸与 Microsoft 研究院的 Antonio Criminisi 交谈。他的想法是,这个项目的秘密武器不应该只由人工智能组成。例如,他的 InnerEye 项目除了利用了人工智能外,还用到了经典(不是基于机器学习)的计算机视觉来分析放射图像。
从某种程度上来说,这可能和你创办人工智能初创公司的初衷不一样。不过,将数据扔到模型并看到它工作的能力还是非常有吸引力的。然而,传统的软件组件更难重现,因为这种组件往往需要程序员思考算法,并利用一些难以获得的领域知识才能构建。
人工智能最好像杠杆一样使用
对业务进行分类的一种方法是,看它是直接增加价值,还是为某些其他价值来源提供杠杆作用。让我们以一家电子商务公司为例。如果你创建了新的产品系列,那么你可以做到直接增加价值。以前什么都没有,现在有了小商品,客户就可以为它们支付费用。另一方面,建立新的分销渠道相当于起到杠杆作用。比如,通过开始在 Amazon 上销售你的小商品,你就可以将销售量翻倍。削减成本也是一种杠杆,如果你与中国的小商品供应商达成更好的交易谈判,那么,你的毛利率将会翻一番。
相比直接施力,杠杆更有可能推得更远。但是,杠杆只有在与直接价值来源耦合时才会起作用。如果你将微小的数进行加倍,它就不会停止变小。如果你没有小商品出售的话,那么,获得新的分销渠道就是浪费时间。
在这种情况下,我们应该如何看待人工智能呢?有很多公司试图将人工智能作为它们的直接产品(如用于图像识别的 API 等)。如果你是人工智能专家,那么这个想法可能非常诱人。然而,这实在是一个非常槽糕的主意。首先,你是在与 Google、Amazon 等公司竞争。其次,制造真正有用的通用人工智能产品是非常困难的。比如,我一直想使用 Google 的 Vision API。 不幸的是,我们还没有遇到这样的一个客户:他的需求与我们的产品完全匹配。它要么是太多,要么是不够,总是这样。定制开发可比在圆孔中钉入方形桩钉要好多了。
综上所述,我们可以得出一个结论:将人工智能视为杠杆是更好的选择。你可以采用现有的、可行的商业模式,并通过人工智能来增强它。例如,如果你有个流程依赖于人类的认识劳动力,那么,将这一流程自动化可以提高你的毛利率。我能想到的一些例子是心电图分析、工业检查、卫星图像分析等等。同样令人兴奋的是,因为人工智能留在后端,所以你有一些非 AI 选择来形成并保持你的竞争优势。
结论:真正重要的是比对手拥有更好的数据
人工智能是一种真正的革命性技术。但是,将你的初创公司建立在人工智能之上可是一件非常棘手的事情。你不应该仅仅依赖于你的人工智能技能,因为,它们会因更大的市场趋势而贬值。
构建人工智能模型可能是一件非常有趣的事情,但真正重要的事情是拥有比竞争对手更好的数据。要知道,保持住竞争优势是很难的事情,特别是如果遇到的是比你更有钱的竞争对手时,而你刚有了人工智能的点子,那么这种情况很可能会发生。
因此,你的目标应该是创建一个可扩展的数据收集过程,这个过程很难被竞争对手复制。人工智能非常适合颠覆依赖低资质人员认知工作的行业,因为它允许自动化这类工作。