为了更好的了解社区的AI+量化项目,在这里介绍一个ChatGPT与交易的可能结合最简略版本:利用ChatGPT o1和Multi-Agent开发的交易策略。本篇文章旨在展示如何让多个智能Agent协同工作,完成复杂的金融数据分析、策略开发和风险管理,让不懂量化的同学也能够上手实践感受AI的强大能力。探索AI与量化结合的最新前沿,欢迎关注公众号加入LLMQuant社区。
背景介绍
在快速变化的金融市场中,实时分析和决策变得至关重要。传统的单一模型往往无法满足这种需求,因此,我们引入了Multi-Agent系统,每个Agent都有特定的角色和任务,通过协作完成整体目标。
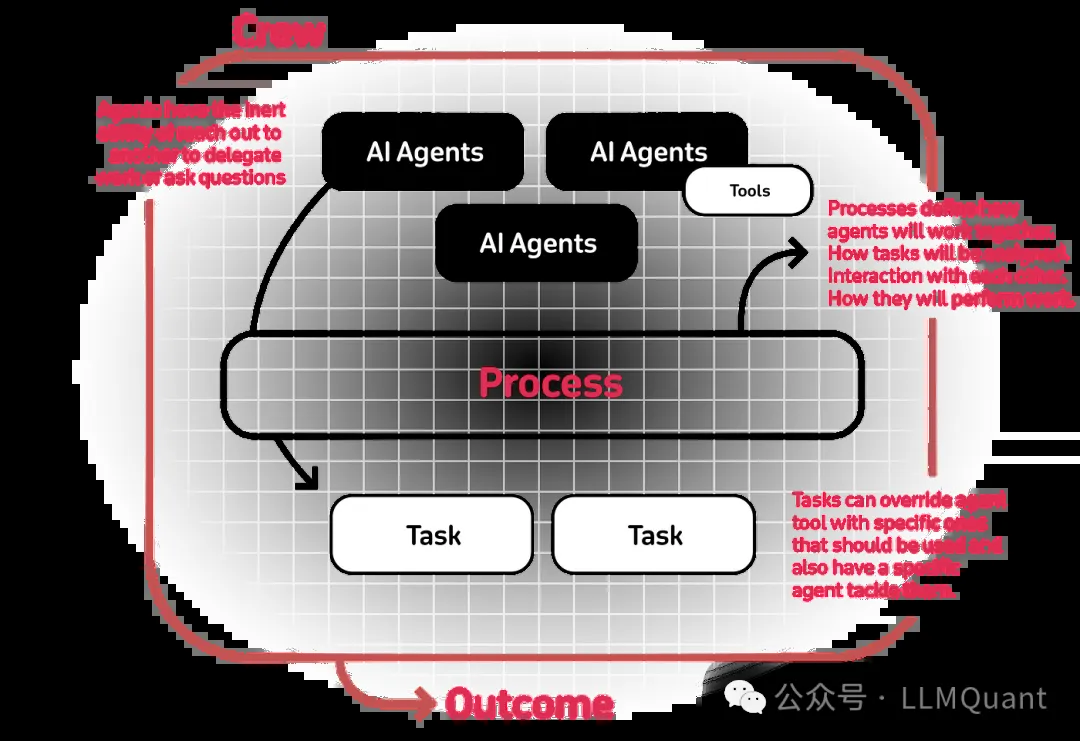
系统架构
我们设计了以下四个智能Agent:
1. 数据分析Agent(Data Analyst Agent)
• 职责: 实时监控和分析市场数据,识别趋势,预测市场走势。
• 特点: 擅长金融市场的数据统计和机器学习,是交易决策的重要基础。
2. 交易策略开发Agent(Trading Strategy Developer Agent)
• 职责: 基于数据分析Agent的洞察,开发和测试交易策略。
• 特点: 深入理解金融市场和定量分析,致力于制定最有利可图且风险最低的策略。
3. 交易顾问Agent(Trade Advisor Agent)
• 职责: 根据批准的交易策略,建议最佳的交易执行方案。
• 特点: 专注于交易的时间、价格和执行细节,确保交易高效且符合策略。
4. 风险管理Agent(Risk Advisor Agent)
• 职责: 评估潜在交易的风险,提供详细的风险分析和缓解建议。
• 特点: 精通风险评估模型和市场动态,确保交易活动符合公司的风险容忍度。
此外,我们还引入了一个管理者(Manager),由 ChatGPT o1 驱动,负责协调所有Agent的工作,确保任务的高效分配和协作。
工作流程
整个系统的工作流程如下:
1. 数据收集与分析:数据分析Agent持续监控选定股票(例如 AAPL)的市场数据,利用统计模型和机器学习算法,识别市场趋势并预测未来走势。
2. 策略开发:交易策略开发Agent基于数据分析Agent的洞察,以及用户定义的风险容忍度和交易偏好,开发和优化交易策略。
3. 执行计划:交易顾问Agent分析批准的策略,考虑当前市场条件,制定最佳的交易执行计划,建议何时以及如何执行交易。
4. 风险评估:风险管理Agent评估拟议交易的潜在风险,提供详细的风险分析报告,并建议风险缓解措施。
5. 任务协调:管理者Agent协调上述所有步骤,确保各Agent之间的信息流畅,任务有效衔接。
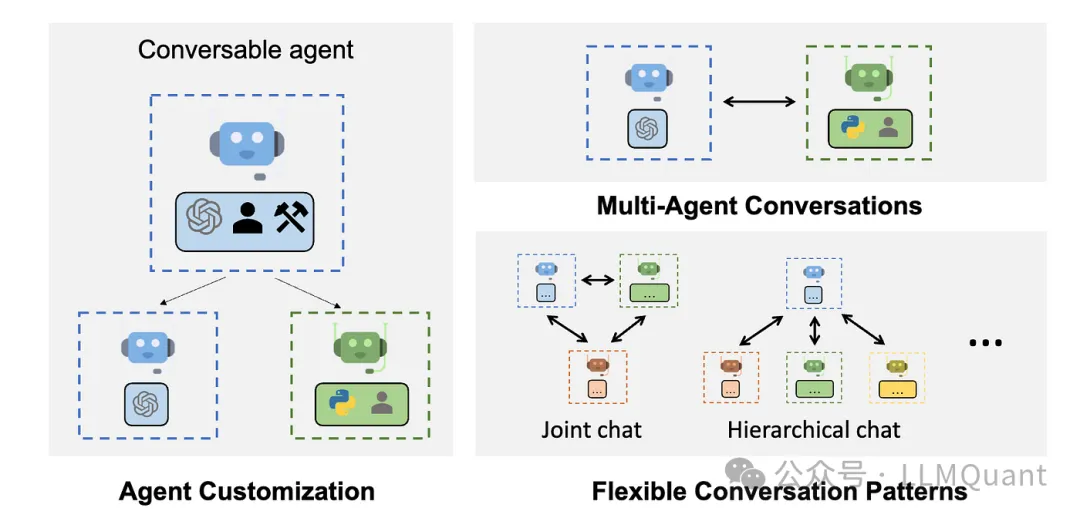
代码实现
下面,我们将展示如何使用 Python 和相关库来实现这个Multi-Agent系统。
环境配置
首先,确保安装必要的库:
!pip install crewai==0.28.8 crewai_tools==0.1.6 langchain_community==0.0.29
导入库和设置 API 密钥
import os
from crewai import Agent, Task, Crew
from crewai_tools import ScrapeWebsiteTool, SerperDevTool
from langchain_openai import ChatOpenAI
# 获取 API 密钥(假设已存储在环境变量中)
openai_api_key = os.getenv("OPENAI_API_KEY")
os.environ["OPENAI_MODEL_NAME"] = 'gpt-3.5-turbo' # 或者 'gpt-4',取决于您的权限
os.environ["SERPER_API_KEY"] = os.getenv("SERPER_API_KEY")
初始化工具
search_tool = SerperDevTool()
scrape_tool = ScrapeWebsiteTool()
创建智能Agent
1. 数据分析Agent
data_analyst_agent = Agent(
role="Data Analyst",
goal="Monitor and analyze market data in real-time to identify trends and predict market movements.",
backstory="Specializing in financial markets, this agent uses statistical modeling and machine learning to provide crucial insights.",
verbose=True,
allow_delegation=True,
tools=[scrape_tool, search_tool]
)
2. 交易策略开发Agent
trading_strategy_agent = Agent(
role="Trading Strategy Developer",
goal="Develop and test various trading strategies based on insights from the Data Analyst Agent.",
backstory="Equipped with a deep understanding of financial markets and quantitative analysis, this agent devises and refines trading strategies.",
verbose=True,
allow_delegation=True,
tools=[scrape_tool, search_tool]
)
3. 交易顾问Agent
execution_agent = Agent(
role="Trade Advisor",
goal="Suggest optimal trade execution strategies based on approved trading strategies.",
backstory="This agent specializes in analyzing the timing, price, and logistical details of potential trades.",
verbose=True,
allow_delegation=True,
tools=[scrape_tool, search_tool]
)
4. 风险管理Agent
risk_management_agent = Agent(
role="Risk Advisor",
goal="Evaluate and provide insights on the risks associated with potential trading activities.",
backstory="Armed with a deep understanding of risk assessment models and market dynamics, this agent scrutinizes the potential risks of proposed trades.",
verbose=True,
allow_delegation=True,
tools=[scrape_tool, search_tool]
)
创建任务
1. 数据分析任务
data_analysis_task = Task(
description=(
"Continuously monitor and analyze market data for the selected stock({stock_selection}). "
"Use statistical modeling and machine learning to identify trends and predict market movements."
),
expected_output=(
"Insights and alerts about significant market opportunities or threats for {stock_selection}."
),
agent=data_analyst_agent,
)
2. 策略开发任务
strategy_development_task = Task(
description=(
"Develop and refine trading strategies based on the insights from the Data Analyst and user-defined risk tolerance({risk_tolerance}). "
"Consider trading preferences({trading_strategy_preference})."
),
expected_output=(
"A set of potential trading strategies for {stock_selection} that align with the user's risk tolerance."
),
agent=trading_strategy_agent,
)
3. 执行计划任务
execution_planning_task = Task(
description=(
"Analyze approved trading strategies to determine the best execution methods for {stock_selection}, "
"considering current market conditions and optimal pricing."
),
expected_output=(
"Detailed execution plans suggesting how and when to execute trades for {stock_selection}."
),
agent=execution_agent,
)
4. 风险评估任务
risk_assessment_task = Task(
description=(
"Evaluate the risks associated with the proposed trading strategies and execution plans for {stock_selection}. "
"Provide a detailed analysis of potential risks and suggest mitigation strategies."
),
expected_output=(
"A comprehensive risk analysis report detailing potential risks and mitigation recommendations for {stock_selection}."
),
agent=risk_management_agent,
)
创建管理者和组建团队
financial_trading_crew = Crew(
agents=[
data_analyst_agent,
trading_strategy_agent,
execution_agent,
risk_management_agent
],
tasks=[
data_analysis_task,
strategy_development_task,
execution_planning_task,
risk_assessment_task
],
manager_llm=ChatOpenAI(model="gpt-3.5-turbo", temperature=0.7),
process=Process.hierarchical,
verbose=True
)
运行系统
我们可以提供一些输入参数来启动流程:
financial_trading_inputs = {
'stock_selection':'AAPL',
'initial_capital':'100000',
'risk_tolerance':'Medium',
'trading_strategy_preference':'Day Trading',
'news_impact_consideration':True
}
启动系统:
result = financial_trading_crew.kickoff(inputs=financial_trading_inputs)
显示最终结果:
from IPython.display import Markdown
Markdown(result)
回测演示
在运行上述代码后,各个Agent将协同工作,完成对 AAPL 股票的实时数据分析、策略开发、执行计划和风险评估。最终结果将以 Markdown 格式呈现,包括对市场的洞察、建议的交易策略、执行计划和风险分析报告。欢迎使用回测平台观察交易效果。
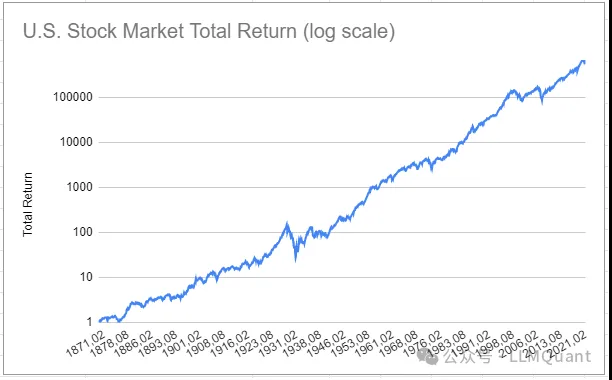
挑战与解决方案
1. 数据质量和可用性
• 挑战: 市场数据可能不完整或存在延迟。
• 解决方案: 使用多个可靠的数据源,并实现数据清洗和验证机制。
2. 实时处理能力
• 挑战: 需要快速处理大量数据,实时生成洞察。
• 解决方案: 优化算法,利用并行处理和高性能计算资源。
3. 风险管理复杂性
• 挑战: 市场风险多变,难以全面评估。
• 解决方案: 引入高级风险评估模型,持续更新风险参数。
4. Agent协调
• 挑战: 多个Agent之间的通信和协作可能出现问题。
• 解决方案: 使用强大的管理者Agent(ChatGPT o1)来协调任务,确保信息流畅。
结论与未来展望
通过本篇文章,我们展示了Multi-Agent系统在金融分析中的巨大潜力。各个Agent分工明确,协同工作,实现了从数据分析到策略开发、执行计划和风险评估的全流程自动化。
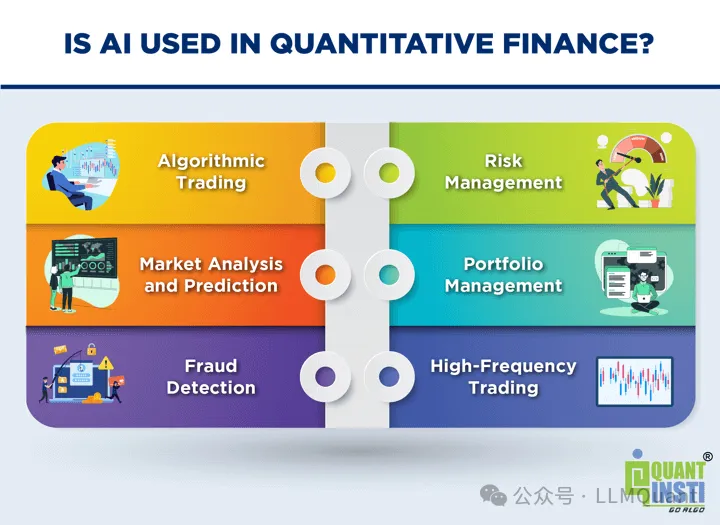
未来可能改进的方向包括:
• 扩展到更多市场和资产类别: 包括外汇、期货和加密货币等。
• 引入更先进的模型和算法: 提高预测准确性和策略有效性。
• 优化用户体验: 开发可视化界面,方便用户与系统交互。
• 加强风险管理: 增加情景分析和压力测试功能,提升风险评估水平。
希望本篇文章能为大家带来AI+Quant的启发,欢迎加入LLMQuant社区获得更加成熟交流和探讨!